最近开始研究如何对手机应用中的识别算法进行加速,搜索后发现了小米的MACE。
Mobile AI Compute Engine (MACE) 是一个专为移动端异构计算设备优化的深度学习前向预测框架。 覆盖了常见的移动端计算设备(CPU,GPU和DSP)。支持的硬件加速比较全面,是小米提供的一个比较有诚意的框架。
这篇博文主要记录一下环境的配置,以及把小米提供的例子运行一下,以这样一个过程来熟悉使用方法。当然大家看MACE的官方文档
MACE也可以获得这些知识,不过我会对我在使用过程中遇到的一些坑进行记录。
环境配置
环境配置有两种方式,一种是自己安装环境,并不是太复杂,一种是使用小米提供的docker,很简单,但是需要对docker有基本的了解。
1.使用docker的环境(推荐)
小米提供了两种docker,一个是little edition,一个是full edition,一般使用little edition已经足够了, full edition版本提供了多个版本的android NDK。
使用docker pull将image拉下来
docker pull registry.cn-hangzhou.aliyuncs.com/xiaomimace/mace-dev-lite
然后就可以启动container了
# Create a container named `mace-dev`
docker run -it --privileged -d --name mace-dev
-v /dev/bus/usb:/dev/bus/usb --net=host
-v /local/path:/container/path
-v /usr/bin/docker:/usr/bin/docker
-v /var/run/docker.sock:/var/run/docker.sock
registry.cn-hangzhou.aliyuncs.com/xiaomimace/mace-dev-lite
# Execute an interactive bash shell on the container
docker exec -it mace-dev /bin/bash
使用docker的image非常方便,我们不用自己配置环境,只是下载image需要花费一些时间。
2.自己安装环境
必须的依赖环境如下:
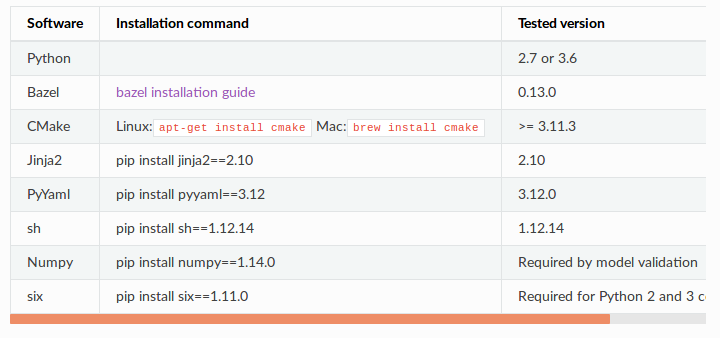
安装依赖的时候最好使用测试过的版本号,否则可能存在编译不过的情况。
安装bazel
export BAZEL_VERSION=0.13.1
mkdir /bazel &&
cd /bazel &&
wget https://github.com/bazelbuild/bazel/releases/download/$BAZEL_VERSION/bazel-$BAZEL_VERSION-installer-linux-x86_64.sh &&
chmod +x bazel-*.sh &&
./bazel-$BAZEL_VERSION-installer-linux-x86_64.sh &&
cd / &&
rm -f /bazel/bazel-$BAZEL_VERSION-installer-linux-x86_64.sh
我之前bazel安装成了0.21.0,就会导致编译的时候报下面的错误:
ERROR: Analysis of target '//mace/libmace:libmace_dynamic' failed; build aborted: no such package '@tflite//': The native http_archive rule is deprecated. load("@bazel_tools//tools/build_defs/repo:http.bzl", "http_archive") for a drop-in replacement.
如果已经遇到了这个问题,将bazel版本回退就好了,最好是用验证过的版本0.13.0。bazel的回退其实很简单,只需要按照上面的安装命令重新安装就可以了。
安装NDK
# Download NDK r15c
cd /opt/ &&
wget -q https://dl.google.com/android/repository/android-ndk-r15c-linux-x86_64.zip &&
unzip -q android-ndk-r15c-linux-x86_64.zip &&
rm -f android-ndk-r15c-linux-x86_64.zip
export ANDROID_NDK_VERSION=r15c
export ANDROID_NDK=/opt/android-ndk-${ANDROID_NDK_VERSION}
export ANDROID_NDK_HOME=${ANDROID_NDK}
# add to PATH
export PATH=${PATH}:${ANDROID_NDK_HOME}
安装其他tools
apt-get install -y --no-install-recommends
cmake
android-tools-adb
pip install -i http://pypi.douban.com/simple/ --trusted-host pypi.douban.com setuptools
pip install -i http://pypi.douban.com/simple/ --trusted-host pypi.douban.com
"numpy>=1.14.0"
scipy
jinja2
pyyaml
sh==1.12.14
pycodestyle==2.4.0
filelock
安装tensorflow
pip install -i http://pypi.douban.com/simple/ --trusted-host pypi.douban.com tensorflow==1.8.0
编译使用
1.下载MACE代码
git clone https://github.com/XiaoMi/mace.git
cd mace/
git fetch --all --tags --prune
2.下载models
git clone https://github.com/XiaoMi/mace-models.git
models中是小米已经训练过的一些models,刚开始学习的时候可以使用这些models进行试验。
模型转换
1.编译mace libs
可以使用下面的命令自己编译,也可以从mace网站上下载已经编译好的libs。
cd path/to/mace
# Build library
# output lib path: builds/lib
bash tools/build-standalone-lib.sh
最后我们在mace目录下会得到一个builds文件夹,有一个include文件夹和一个libs文件夹,主要是为不同的环境生成cpu gpu优化的静态和动态库。
├── include
│ └── mace
│ └── public
│ └── mace.h
└── lib
├── aarch64_linux_gnu
│ └── cpu_gpu
│ ├── libmace.a
│ └── libmace.so
├── arm64-v8a
│ └── cpu_gpu
│ ├── libmace.a
│ └── libmace.so
├── armeabi-v7a
│ ├── cpu_gpu
│ │ ├── libmace.a
│ │ └── libmace.so
│ └── cpu_gpu_dsp
│ ├── libhexagon_controller.so
│ ├── libmace.a
│ └── libmace.so
├── arm_linux_gnueabihf
│ └── cpu_gpu
│ ├── libmace.a
│ └── libmace.so
└── linux-x86-64
├── libmace.a
└── libmace.so
2.将mobilenetv2转成mace的格式
因为后面我们需要讲生成的文件在android代码中使用,所以需要修改mobilenet-v2.yml和mobilenet-v2-host.yml这两个文件中的
model_graph_format: file
model_data_format: file
更改为
model_graph_format: code
model_data_format: code
然后用convertor脚本开始转换。
cd path/to/mace
# Build library
python tools/converter.py convert --config=/path/to/mace-models/mobilenet-v2/mobilenet-v2.yml
converter.py这个脚本是根据mobilenet-v2.yml中的配置进行转换的,如果需要转换自己的模型文件,就需要写自己的yml文件。
转换成功后得到了mobilenet-v2文件夹,其中mobilenet_v2_index.html是网络结构列表。
......
├── mobilenet-v2
│ ├── include
│ │ └── mace
│ │ └── public
│ │ ├── mace_engine_factory.h ------引用模型时需要包含的h文件
│ │ └── mobilenet_v2.h ------引用模型时需要包含的h文件
│ └── model
│ ├── arm64-v8a
│ │ └── mobilenet-v2.a ------模型库文件
│ └── armeabi-v7a
│ └── mobilenet-v2.a
└── mobilenet_v2_index.html
3.在手机中运行
上面的编译生成的库文件需要在代码中调用,但是如果要方便的跑android demo可以直接在mace/examples/android下跑脚本:
./build.sh dynamic
这个脚本会直接从编译libmace开始开始直到编译出apk文件。
编译完成后安装apk
adb install ./app/build/outputs/apk/app/release/app-app-release.apk
使用GPU计算大约会比CPU提升一倍的速度。
下一篇介绍如何使用mace对自己的模型加速。